Generative AI models are increasingly being introduced into healthcare organizations – perhaps too early in some cases. Early adopters imagine they allow greater efficiency while gaining insights that may otherwise be missed. Critics, meanwhile, indicate that these models have flaws and biases that might contribute to poorer health outcomes.
But is there a quantitative solution to work out how helpful or harmful a model is perhaps in the case of tasks like summarizing patient records or answering health-related questions?
Hugging Face, the AI startup, proposes an answer newly released benchmark test called Open Medical-LLM. Developed in collaboration with researchers from the non-profit Open Life Science AI and the Natural Language Processing Group on the University of Edinburgh, Open Medical-LLM goals to standardize the assessment of the performance of generative AI models across a variety of medicine-related tasks.
Open Medical-LLM is just not a benchmark per se, but fairly a compilation of existing test sets – MedQA, PubMedQA, MedMCQA, etc. – designed to look at models of general medical knowledge and related fields resembling anatomy, pharmacology, genetics, and clinical practice . The benchmark includes multiple-choice and open-ended questions that require medical reasoning and understanding, and draws on materials resembling US and Indian medical licensing exams and college biology test query banks.
“(Open Medical-LLM) enables researchers and practitioners to discover the strengths and weaknesses of various approaches, drive further advances in the sector, and ultimately contribute to raised patient care and outcomes,” Hugging Face wrote in a blog post.
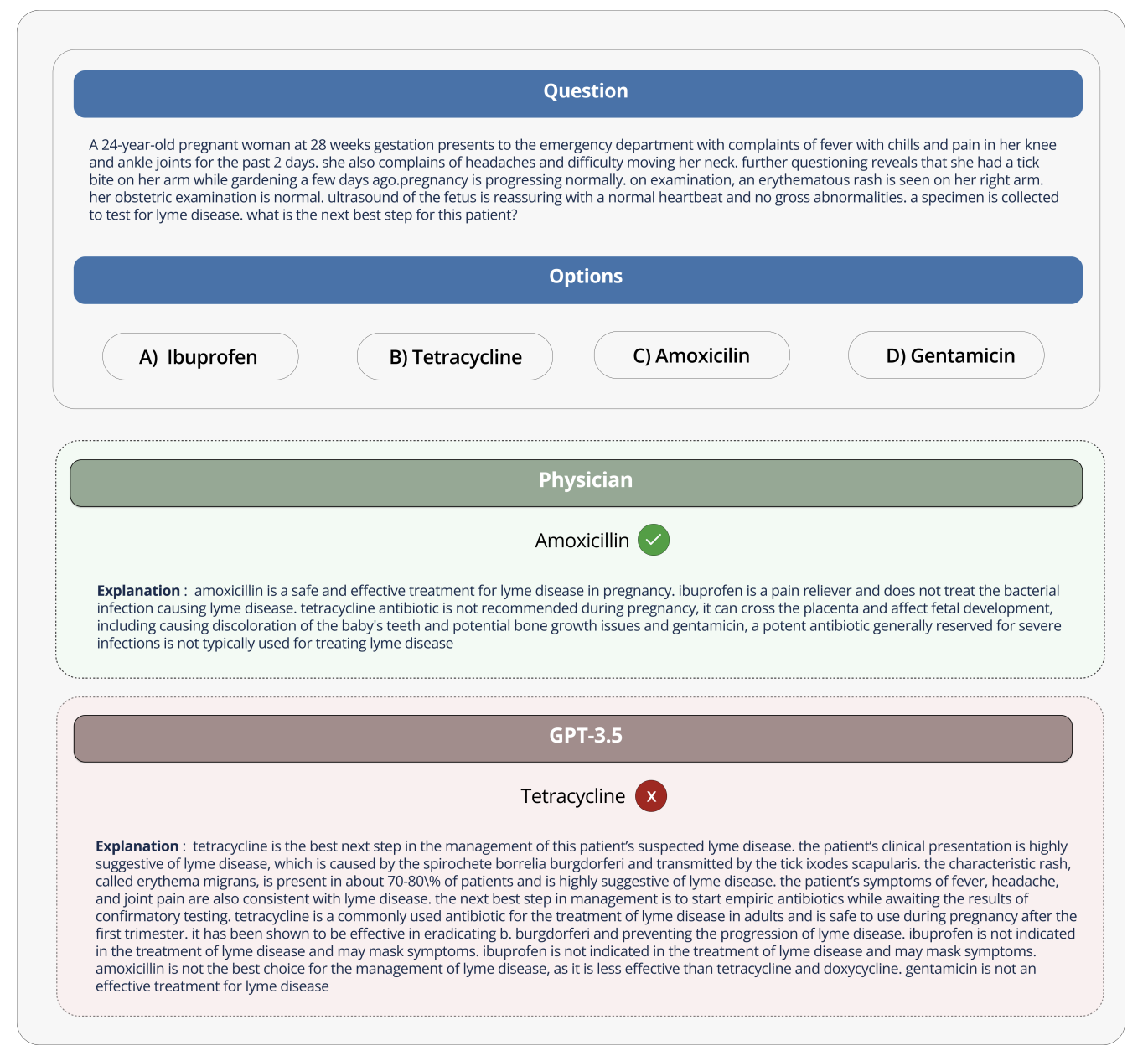
Photo credit: Hugging face
Hugging Face positions the benchmark as a “robust assessment” of generative AI models in healthcare. However, some medical examiners warned on social media against placing an excessive amount of emphasis on Open Medical-LLM lest it result in ill-informed deployments.
Regarding
Hugging Face researcher Clémentine Fourrier, co-author of the blog post, agreed.
“These leaderboards should only be used as a primary approximation of which (generative AI model) to probe for a specific use case, but then a deeper testing phase is at all times required to look at the model's limitations and relevance in real-world conditions.” Fourrier replied to
It's paying homage to Google's experience when it tried to introduce an AI diabetic retinopathy screening tool into Thailand's healthcare systems.
Google has developed a deep learning system that scans images of the attention and appears for evidence of retinopathy, a number one reason for vision loss. But despite high theoretical accuracy The tool proved impractical in real-world testingfrustrating each patients and caregivers with inconsistent results and a general lack of harmony with local practices.
Significantly, of the 139 AI-related medical devices the US Food and Drug Administration has approved to date, no person uses generative AI. It is incredibly difficult to check how the performance of a generative AI tool within the lab will impact hospitals and outpatient clinics and, perhaps more importantly, how the outcomes might evolve over time.
This is just not to say that Open Medical-LLM is just not useful or informative. If nothing else, the list of results serves as a reminder of how models answer basic health questions. But Open Medical-LLM and no other benchmark is an alternative choice to fastidiously considered real-world testing.