The potential of using artificial intelligence in drug discovery and development has been raised each excitement and skepticism amongst scientists, investors and most people.
“Artificial intelligence is Taking over drug development“, claim some firms and researchers. In recent years, interest in using AI to develop drugs and optimize clinical trials has led to a rise in research and investment. AI-driven platforms like AlphaFoldwhich won the Nobel Prize in 2024 for its ability to predict the structure of proteins and design recent ones, shows the potential of AI to speed up drug development.
AI in drug research is “nonsense”.“ warn some industry veterans. They argue that “AI’s potential to speed up drug discovery requires….” Reality check“As AI-generated drugs usually are not yet capable of handle the 90 percent failure rate of recent drugs in clinical trials. In contrast to the success of AI in Image evaluationits impact on drug development stays unclear.
nortonrsx/iStock via Getty Images Plus
We have been tracking the usage of AI in drug development in our work as Pharmaceutical scientist each in science and within the pharmaceutical industry and as former program manager within the Defense Advanced Research Projects Agency (DARPA). We argue that AI in drug development shouldn’t be yet a game-changer neither is it complete nonsense. AI shouldn’t be a black box that may turn any idea into gold. Rather, we view it as a tool that, when used properly and competently, can assist address the basis causes of drug failure and streamline the method.
Most work on using AI in drug development goals to cut back the money and time required to bring a drug to market – currently 10 to fifteen years and $1 to $2 billion. But can AI really revolutionize drug development and improve success rates?
AI in drug development
Researchers have applied AI and machine learning to it Every stage the drug development process. These include, but usually are not limited to, identifying targets within the body, screening potential candidates, developing drug molecules, predicting toxicity, and choosing patients who might respond best to the drugs in clinical trials.
Between 2010 and 2022 20 AI-focused startups discovered 158 drug candidates, 15 of which entered clinical trials. Some of those drug candidates were capable of complete preclinical testing within the laboratory and enter human trials in only 30 months compared to traditional drugs 3 to six years. This success demonstrates the potential of AI to speed up drug development.
On the opposite hand, while AI platforms can quickly discover compounds that act on cells in a petri dish or in animal models, the success of those candidates in clinical trials – where most drug failures occur – stays highly uncertain.
Unlike other fields where large, high-quality data sets can be found to coach AI models akin to image evaluation and language processing, AI in drug development has limitations small, low quality data sets. It is difficult to create drug-related cellular, animal, or human datasets for tens of millions to billions of compounds. While AlphaFold is a breakthrough in protein structure prediction, how precise It stays uncertain whether it concerns the drug design. Minor changes to the structure of a drug can greatly affect its activity within the body and subsequently its effectiveness in treating disease.
Survival bias
Like AI, previous innovations in drug development like Computer-aided drug designThe Human genome project and high-throughput screening have improved individual steps of the method over the past 40 years, but drug failure rates haven’t improved.
Most AI researchers can handle certain tasks within the drug development process in the event that they have high-quality data and specific inquiries to answer. But they often are not acquainted with all the scope of drug development, reducing challenges in pattern recognition problems and refining individual steps of the method. Meanwhile, many scientists with expertise in drug development lack training in AI and machine learning. These communication barriers can prevent scientists from going beyond the mechanisms of current development processes and identifying the basis causes of drug failure.
Current approaches to drug development, including those using AI, can have reached a dead end Survivor bias Trap by focusing an excessive amount of on less critical facets of the method big problems ignored that contribute essentially the most to failure. This is akin to repairing damage to the wings of aircraft coming back from battlefields in World War II, while concurrently neglecting fatal weak points within the engines or cockpits of the aircraft that never returned. Researchers often focus an excessive amount of on the best way to improve the person properties of a drug moderately than on the basis causes of failure.
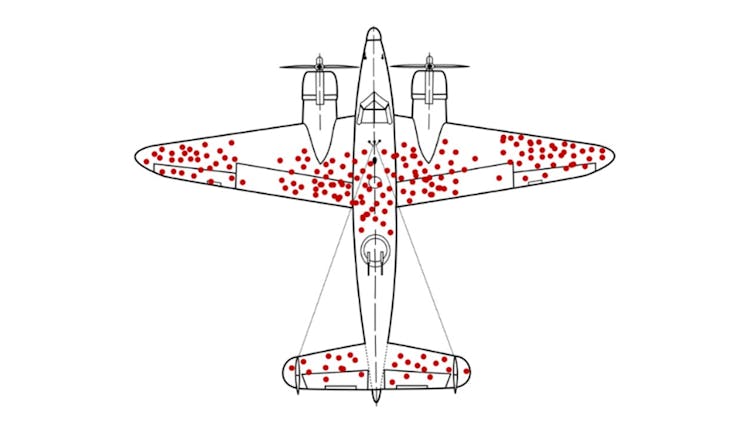
Martin Grandjean, McGeddon, US Air Force/Wikimedia Commons, CC BY-SA
The current drug development process is ongoing like an assembly linebased on a checkbox approach with comprehensive testing at every step of the method. While AI may have the ability to cut back the time and value of the laboratory-based preclinical phases of this assembly line, it’s unlikely to extend success rates within the more costly clinical phases where testing is performed on humans. The persistent 90% failure rate for drugs in clinical trials despite 40 years of process improvements highlights this limitation.
Fighting the causes
The failure of medication in clinical trials shouldn’t be solely resulting from the way in which these trials are designed. Selection of the incorrect drug candidates testing in clinical trials can be a very important factor. New AI-driven strategies could help address each of those challenges.
At the moment, three interdependent aspects Cause of most drug failures: dosage, safety and effectiveness. Some medications fail because they’re too toxic or unsafe. Other medications fail because they’re considered ineffective, actually because the dose can’t be increased further without causing harm.
We and our colleagues propose a machine learning system to support the collection of drug candidates by predicting dosage, Security and effectiveness based on five previously ignored drug characteristics. Specifically, researchers could use AI models to find out how specifically and strongly the drug binds to known and unknown targets, how high these targets are within the body, how concentrated the drug becomes in healthy and diseased tissues, and what structural properties the drug has .
These features of AI-generated drugs could possibly be tested in what we call Phase 0+ trialsusing extremely low doses in patients with severe and mild disease. This could help researchers find optimal drugs while reducing the prices of the present “test-and-see” approach to clinical trials.
While AI alone may not revolutionize drug development, it might probably help address the basis causes of drug failure and streamline the lengthy process leading as much as approval.