A public interest group filed a US federal grievance against artificial intelligence hiring tool HireVuein 2019 for fraudulent hiring practices. The software, adopted by a whole lot of corporations, favored certain facial expressions, speaking styles and voice tones and disproportionately disadvantaged minority candidates.
The Electronic Privacy Information Center argued that HireVue's results were “biased, unprovable and never reproducible.” Although The company has since stopped using facial recognitionConcerns remain about biases in other biometric data, equivalent to speech patterns.
Amazon has also stopped using it AI recruiting toolas reported in 2018 after it was discovered that this was the case biased against women. The algorithm was trained on male-dominated resumes submitted over a 10-year period. preferred male candidates by downgrading applications that contained the word “women” and penalizing graduates of girls’s colleges. The engineers tried to eliminate these prejudices, but couldn’t guarantee neutrality, which led to the cancellation of the project.
These examples illustrate a growing interest in recruitment and selection: while some corporations are Using AI to eliminate human bias in hiringit may often reinforce and strengthen existing inequalities. Given the rapid integration of AI in human resources management In many organizations it is vital to boost awareness of the complex ethical challenges involved.
How AI can create bias
As corporations increasingly depend on algorithms to make necessary hiring decisions, it is vital to pay attention to the next options AI can result in bias in hiring:
1. Bias within the training data. AI systems depend on large data sets – called training data – to learn patterns and make decisions, but their accuracy and fairness are only pretty much as good as the information they’re trained on. If this data comprises historical hiring biases that favor certain demographics, so be it AI will adopt and reproduce the identical biases. For example, Amazon's AI tool was trained on resumes from a male-dominated industry, which resulted in gender bias.
2. Incorrect data collection. Improper data sampling occurs when the information set used to coach an algorithm is just not representative of the broader population it is meant to serve. In the context of hiring, this may occur if Training data overrepresents certain groups – typically white men – while marginalized candidates are underrepresented.
This allows the AI ​​to learn it Favor the characteristics and experiences of the overrepresented group while those from underrepresented groups are penalized or ignored. For example, facial evaluation technologies demonstrably higher error rates for racially motivated peopleparticularly racialized women, as they’re underrepresented in the information used to coach these systems.
3. Feature selection bias. When designing AI systems Developers select specific featuresAttributes or characteristics that must be prioritized or given greater weight in AI decision making. But this one Selected features can result in unfair, biased results and maintain existing inequalities.
For example, AI could give disproportionate value to graduates from prestigious universities, which has been the case prior to now wherein people from privileged backgrounds participate. Or it could prioritize work experiences which are more common amongst certain populations.
This problem is exacerbated when the features chosen are present Proxies for protected characteristicsequivalent to B. Zip code, with which there could also be a detailed connection Race and socioeconomic status attributable to historical residential segregation.
(Shutterstock)
4. Lack of transparency. Many AI systems act as “black boxes,” meaning their decision-making processes are opaque. This lack of transparency makes it difficult for corporations to discover where bias may exist and the way it impacts hiring decisions.
Without insight into how an AI tool makes decisions, it’s difficult to correct biased results or ensure fairness. Both Amazon and HireVue have faced this issue; Users and developers had difficulty understanding how the systems evaluated candidates and why certain groups were excluded.
5. Lack of human supervision. While AI plays a very important role in lots of decision-making processes, it should complement somewhat than replace human judgment. Over-reliance on AI without proper human oversight can result in uncontrolled biases. This problem is exacerbated when recruiters trust AI greater than their very own judgment and imagine within the infallibility of technology.
Overcoming algorithmic bias in hiring
To mitigate these issues, corporations must adopt strategies that prioritize inclusivity and transparency in AI-driven hiring processes. Below are some key solutions to beat AI bias:
1. Diversify training data. One of probably the most effective ways to combat AI bias is thru assurance Training data is comprehensive, diverse and representative a big selection of candidates. This means including data from diverse racial, ethnic, gender, socioeconomic and academic backgrounds.
2. Conduct regular bias audits. Frequent and thorough audits of AI systems must be conducted to discover patterns of bias and discrimination. This includes examining the outcomes of the algorithm, the decision-making processes and its impact on different demographic groups.
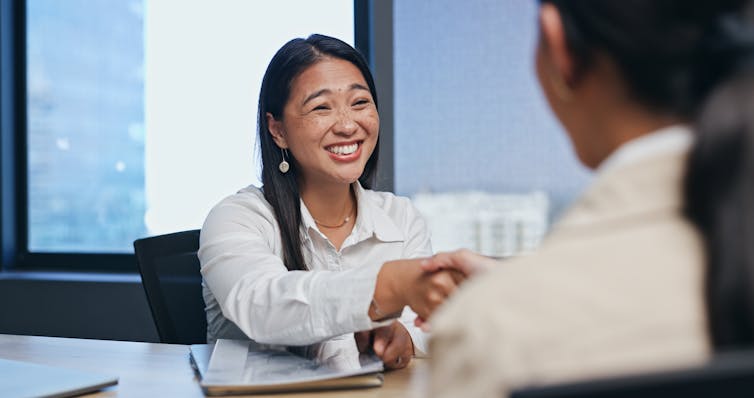
(Shutterstock)
3. Implement fairness-conscious algorithms. Use AI software that respects and is fairness constraints Designed to account for and mitigate bias through balanced outcomes for underrepresented groups. This can include incorporating fairness metrics equivalent to equity, modifying training data to point out less bias, and adjusting model predictions based on fairness criteria to extend equity.
4. Increase transparency. Look for AI solutions that provide insights into their algorithms and decision-making processes to assist discover and eliminate potential biases. Also, ensure that is the case Disclose any use of AI within the hiring process to candidates to take care of transparency together with your applicants and other stakeholders.
5. Maintain human supervision. To maintain control over hiring algorithms, managers and executives must actively review AI-driven decisions, especially when making final hiring decisions. New research highlights the critical role of human oversight Protection against the risks provided by AI applications. However, for this oversight to be effective and meaningful, leaders must make sure that ethical considerations are a part of the hiring process and promote the responsible, inclusive and ethical use of AI.
Bias in algorithm hiring raises serious ethical concerns and requires greater attention to the mindful, responsible and inclusive use of AI. To ensure fairer hiring outcomes and forestall technology from reinforcing systemic bias, it is vital to know and address the moral considerations and biases of AI-driven hiring.