In an ambitious effort to combat the harm attributable to false content social media And News web sitesData scientists are getting creative.
They were still of their training wheels large language models (LLMs) used to create chatbots corresponding to ChatGPT are recruited for detection Fake news. With higher detection, AI systems designed to envision fake news may have the option to warn of and ultimately counteract serious harm Deepfakes, propaganda, Conspiracy theories And Misinformation.
The next level of AI tools will personalize the detection of false content and protect us from it. For this ultimate leap into user-centered AI, data science must draw on behavioral and neuroscience.
Recent work suggests this will be the case don't all the time know consciously that we come across fake news. Neuroscience helps to search out out what goes on unconsciously. Biomarkers corresponding to heart rate, Eye movements And Brain activity) appear to subtly change in response to fake and real content. In other words, these biomarkers will be “tells” that indicate whether we now have been included or not.
For example, when people take a look at faces, eye tracking data shows that we search for blink and blink frequencies Changes in skin color attributable to blood flow. If such elements appear unnatural, it may possibly help us resolve that we’re coping with a deepfake. This knowledge can provide AI a bonus – we will, amongst other things, train it to mimic what humans are in search of.
The personalization of an AI fake news checker is finished through the use of insights from Movement data of the human eye And electrical brain activity that shows which forms of false content have the best neural, psychological and emotional impact, and for whom.
Knowledge of our specific interests, personality and emotional reactionsAn AI fact-checking system could detect and predict which content would trigger the strongest response in us. This could help discover when persons are being tricked and what variety of material fools them most easily.
Counteract damage
Next, the protective measures should be adjusted. To protect ourselves from the harms of pretend news, we also have to develop systems that may intervene – in some form Digital countermeasure against fake news. There are several ways to do that, corresponding to warning labels, links to expert-approved, credible content, and even encouraging people to contemplate different perspectives when reading.
Our own personalized AI fake news checker could possibly be designed to offer each of us with certainly one of these countermeasures to eliminate the damage attributable to incorrect online content.
Such technology is already being tested. Researchers within the USA have studied how people interact with one another a personalised AI fake news checker for social media posts. It has learned to scale back the variety of posts in a newsfeed to the number it believes to be true. As a proof of conceptIn one other study using social media posts, additional news content was added to every media post to encourage users to contemplate alternative perspectives.
Precise detection of pretend news
But whether this all sounds impressive or dystopian, before we get carried away, it could be value asking a couple of basic questions.
Much, if not all, of the work Fake news, deepfakes, disinformation And Misinformation highlights the identical problem any lie detector would face.
There are many forms of lie detectors, not only the lie detector test. Some rely solely on linguistic evaluation. Others are systems designed to read people's faces to see in the event that they reveal micro-emotions that reveal they’re lying. There are also AI systems which can be imagined to recognize whether a face is real or a fake.
Before the detection begins, all of us have to agree on what a lie looks like with the intention to recognize it. Actually in Deception research shows that it may possibly be easier because you may tell people when to lie and when to inform the reality. And so you may have a probability to experience the essential truth in front of you train an individual or a machine to inform the difference because they’re supplied with examples on which to base their judgments.
How good an experienced lie detector is is dependent upon how often he detects a lie when there may be one (hits). But also that they don't often give the impression that somebody is telling the reality once they have actually lied (Miss). This means they should know what the reality is once they see it (true rejection) and never accuse someone of lying regardless that they were telling the reality (false alarm). This refers to signal detection, and the identical logic applies to this Fake news detection which you’ll see within the diagram below.
In order for an AI system to detect fake news very accurately, the hits should be very high (e.g. 90%), the false reports must subsequently be very low (e.g. 10%) and the false alarms must remain low (e.g. B. 10). %), which implies that real news just isn’t called fake. When an AI fact-checking system or a human system based on signal detection is really helpful to us, we will higher understand how good it’s.
It is probably going that there are cases, as recently reported Opinion pollwhere the message content will not be completely false or completely true, but could also be partially correct. We know this since the speed of reports cycles implies that what is taken into account correct at one time could also be correct later prove to be inaccurate, or vice versa. So a fake news checking system has rather a lot to do.
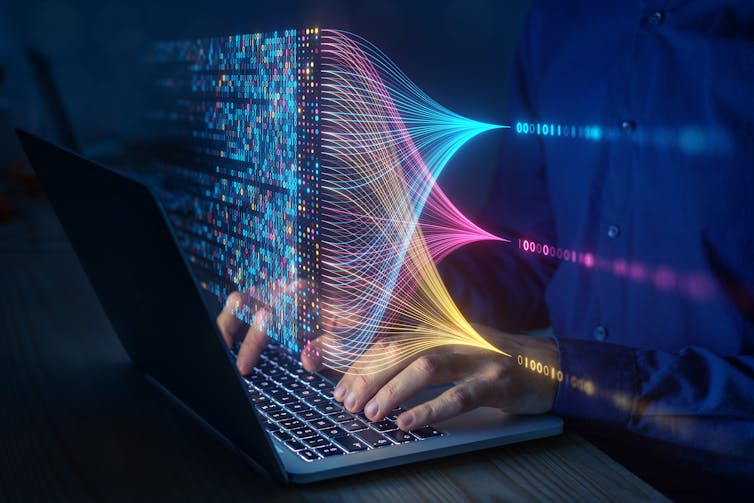
NicoElNino/Shutterstock
If we knew prematurely what was fake and what was real news, how accurately can biomarkers subconsciously indicate which is which? The answer just isn’t very. Neural activity is generally the identical once we come across real and pretend news articles.
When it involves eye tracking studies, it is necessary to know that there are several types of data collected using eye tracking techniques (e.g. the duration our eye fixates on an object, the Frequency with which our eyes move across a visible scene). ).
Depending on what’s being analyzed, some studies show this we draw more attention when displaying incorrect content while others are displaying it Opposite.
Are we there yet?
AI systems available available on the market to detect fake news are already using insights from behavioral science to assist flag and warn us about fake news Contents. So it won't be much of a challenge to have the identical AI systems popping up in our news feeds with protections tailored to our unique user profile. The problem with all of that is that we still have to cover a number of bases to know what works, but in addition to confirm it whether we wish that.
In the worst case scenario, we only see fake news on the web as an excuse to resolve it AI. But false and inaccurate content is all over the place and is being discussed offline. Furthermore, we don't robotically consider all fake news; sometimes we even use it in discussions illustrate bad ideas.
In a possible best-case scenario, data and behavioral sciences are confident concerning the magnitude of the varied harms that fake news could cause. But even here, AI applications combined with scientific wizardry could still be a really poor substitute for less sophisticated but simpler solutions.