A brand new model of artificial intelligence (AI) has just appeared Human-level results achieved on a test measuring “general intelligence.”
On December 20, OpenAI's o3 system reached 85% ARC-AGI benchmarkwell above the previous AI best value of 55% and on par with the common human value. It also performed well on a really difficult math test.
The creation of artificial general intelligence (AGI) is the stated goal of all major AI research laboratories. At first glance, OpenAI seems to have come not less than a big step closer to this goal.
While skepticism stays, many AI researchers and developers feel that something has just modified. For many, the prospect of AGI now seems more real, more urgent and closer than expected. Are you right?
Generalization and intelligence
To understand what the o3 result means, it’s good to understand what the ARC-AGI test is about. Technically, it's a test of an AI system's “example efficiency” in adapting to something recent – what number of examples of a novel situation does the system must see to work out how it really works.
An AI system like ChatGPT (GPT-4) will not be very sample efficient. It was “trained” on thousands and thousands of examples of human text and created probabilistic “rules” about which combos of words are almost certainly.
The result’s pretty good for on a regular basis tasks. It is bad for unusual tasks because there’s less data (less samples) for these tasks.
Bianca De Marchi / AAP
Until AI systems can learn from a small variety of examples and adapt with greater sampling efficiency, they’ll only be used for very repetitive tasks and tasks where occasional errors are tolerable.
The ability to accurately solve previously unknown or novel problems using limited data samples is known as generalization ability. It is widely viewed as a vital, even fundamental, element of intelligence.
Grids and patterns
The ARC-AGI benchmark tests efficient sampling adjustment using small grid square problems like the next. The AI has to work out the pattern that turns the grid on the left into the grid on the precise.
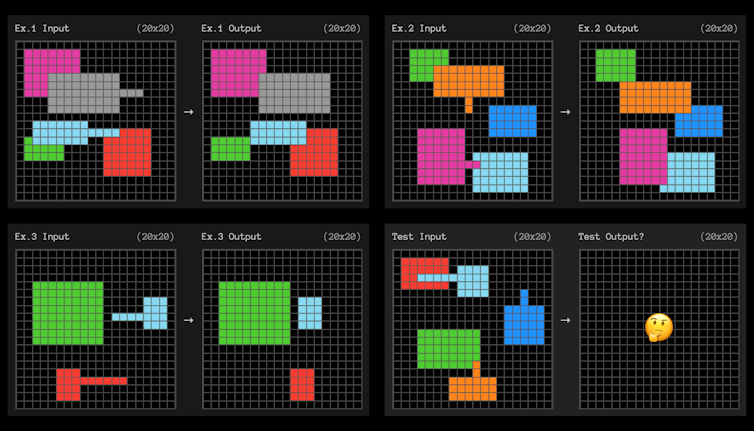
ARC Prize
Each query incorporates three examples so that you can learn from. The AI system then has to work out the principles that “generalize” from the three examples to the fourth.
These are very just like the IQ tests chances are you’ll know from school.
Weak rules and adaptation
We don't know exactly how OpenAI did this, but the outcomes suggest that the o3 model could be very adaptable. Using just a few examples, rules are found that will be generalized.
To work out a pattern, we shouldn't make unnecessary assumptions or be more specific than we actually must be. In theoryIf you may discover the “weakest” rules that do what you wish, then you could have maximized your ability to adapt to recent situations.
What can we mean by the weakest rules? The technical definition is complicated, but weaker rules are frequently those who will be described in simpler statements.
In the instance above, a straightforward English expression of the rule might read something like this: “Any shape with a protruding line moves to the top of that line and 'hides' some other shapes with which it overlaps.”
Looking for thought chains?
Although we don't yet know the way OpenAI achieved this result, it’s unlikely that they intentionally optimized the o3 system to search out weak rules. However, to achieve success within the ARC-AGI tasks, you could have to search out them.
We know that OpenAI began with a general-purpose version of the o3 model (which differs from most other models in that it will possibly spend more time “pondering” about difficult questions) after which adapted it specifically for the ARC-AGI test trained.
French AI researcher Francois Chollet, who designed the benchmark, believes o3 searches through various “thought chains” that describe steps to unravel the duty. It would then select the “best” in response to a loosely defined rule or “heuristic.”
This could be “not dissimilar” to the best way Google's AlphaGo system searched through various possible sequences of moves to beat the Go world champion.
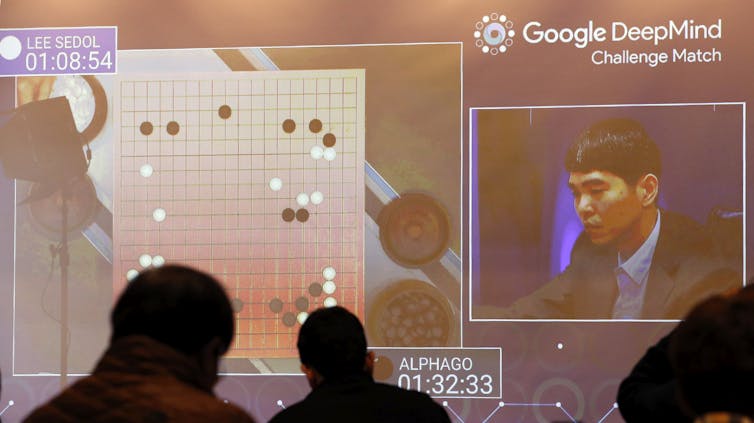
Lee Jin-man / AP
You can imagine these thought chains like programs that fit the examples. If it's like Go-Playing AI, then after all it needs a heuristic or loose rule to make your mind up which program is best.
Thousands of various, seemingly equally valid programs could possibly be generated. This heuristic could possibly be: “Choose the weakest” or “Choose the only”.
However, if it's like AlphaGo, then they simply had an AI create a heuristic. This was the method for AlphaGo. Google trained a model to rate different sequences of movements as higher or worse than others.
What we don't know yet
The query then is, is that this really closer to AGI? If that is how o3 works, the underlying model will not be significantly better than previous models.
The concepts that the model learns from the language will not be more suitable for generalization than before. Instead, we may see a generalizable “chain of thought” found through the extra steps of coaching a heuristic specialized for that test. As all the time, the proof is within the pudding.
Almost every part about o3 stays unknown. OpenAI's disclosure is proscribed to just a few media presentations and early testing to a handful of researchers, labs and AI security institutions.
To truly understand o3's potential, extensive work is required, including evaluations and an understanding of the distribution of its capabilities and the way often it fails and the way often it succeeds.
When o3 is finally released, we'll have a significantly better idea of whether it's about as adaptable as the common human.
If so, it could have enormous, revolutionary economic impact and usher in a brand new era of self-improving accelerated intelligence. We need recent standards for AGI itself and serious consideration of the way it ought to be managed.
If not, it is going to still be a formidable result. However, on a regular basis life will remain largely the identical.