Researchers at Google DeepMind have developed NATURAL PLAN, a benchmark to guage the flexibility of LLMs to plan real-world tasks based on natural language instructions.
The next stage of AI evolution is to depart the confines of a chat platform and tackle agent roles to perform cross-platform tasks for us. But that's harder than it sounds.
Planning tasks like scheduling a gathering or putting together a vacation plan could appear easy to us. Humans are good at pondering through multiple steps and predicting whether or not a plan of action will achieve the specified goal.
This could appear easy to you, but even the most effective AI models have trouble with planning. Could we compare them to see which LLM is best at planning?
The NATURAL PLAN benchmark tests LLMs on three planning tasks:
- Travel planning – Planning a travel route bearing in mind flight and destination restrictions
- Meeting planning – Plan meetings with several friends in numerous locations
- Calendar planning – Planning work meetings between multiple people, bearing in mind existing schedules and various constraints
The experiment began with a choice of just a few trials by which the models were supplied with 5 examples of prompts and the corresponding correct responses. They were then presented with planning prompts of various difficulty.
Here is an example prompt and solution provided to the models for example:
Results
The researchers tested GPT-3.5, GPT-4, GPT-4o, Gemini 1.5 Flash and Twins 1.5 Pro, none of which performed particularly well in these tests.
However, the outcomes should have been well received within the DeepMind office, as Gemini 1.5 Pro emerged because the winner.

As expected, results deteriorated exponentially for more complex prompts where the number of individuals or cities was increased. For example, take a look at how quickly accuracy dropped as more people were added to the meeting planning test.
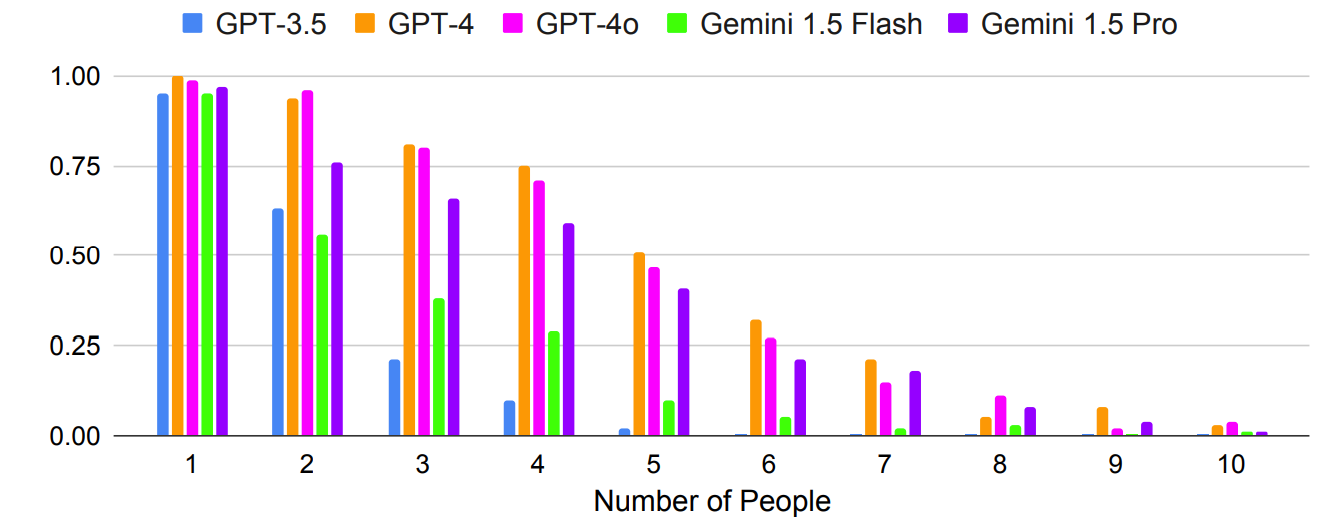
Could multi-shot prompting improve accuracy? Research results suggest that it is feasible, but provided that the model has a sufficiently large context window.
Thanks to the larger context window of Gemini 1.5 Pro, more contextual examples will be used than with the GPT models.
The researchers found that Gemini Pro 1.5's accuracy in trip planning improved from 2.7% to 39.9% when increasing the variety of shots from 1 to 800.
The paper noted, “These results show the promise of contextual planning, where long-term context features enable LLMs to leverage additional context to enhance planning.”
One strange finding was that GPT-4o was really bad at trip planning. The researchers found that it had difficulty “understanding and respecting flight connectivity and travel date constraints.”
Another curious finding was that self-correction resulted in a big drop in performance for all models. When the models were asked to envision their work and make corrections, they made more errors.
Interestingly, the stronger models akin to GPT-4 and Gemini 1.5 Pro suffered greater losses during self-correction than GPT-3.5.
Agentic AI is an exciting prospect and we’re already seeing some practical use cases in Microsoft co-pilot agents.
However, the outcomes of the NATURAL PLAN benchmark tests show that there continues to be an extended strategy to go before AI can handle more complex planning.
The DeepMind researchers concluded that “NATURAL PLAN may be very difficult for contemporary models to unravel.”
It seems that AI is not going to replace travel agents and private assistants yet.