The rapid rise of generative artificial intelligence similar to OpenAI's GPT-4 has brought remarkable progress, but in addition poses significant risks.
One of probably the most pressing problems is model collapse, a phenomenon during which AI models that were largely trained on AI-generated content are inclined to worsen over timeThis degradation occurs when AI models lose information in regards to the actual underlying data distribution, resulting in increasingly similar and fewer diverse results filled with biases and errors.
While the web is flooded with AI-generated real-time content, the dearth of recent human-generated or natural data exacerbates this problem even furtherWithout a gradual flow of diverse, high-quality data, AI systems risk becoming less accurate and reliable.
In view of those challenges, synthetic data has emerged as a promising solution. They are designed to copy the statistical properties of real data as closely as possible and may Providing the amount needed to coach AI models while ensuring the inclusion of a wide range of data points.
(Shutterstock)
Synthetic data doesn’t contain any real or personal information. Instead Computer algorithms use statistical patterns and features observed in real data sets to generate syntheticThese synthetic datasets are tailored to the precise needs of researchers and supply scalable and cost-effective alternatives to traditional data collection.
My research explores the advantages of synthetic data in constructing more diverse and safer AI models, and potentially addresses the risks of model collapse. I also address key challenges and ethical considerations in future synthetic data development.
Use of synthetic data
From training AI models and testing software to making sure privacy in data exchange, the applying areas of artificially generated information that replicates the properties of real data are wide-ranging.
Synthetic data in healthcare helps researchers Analyze patient trends and health outcomesthat support the event of advanced diagnostic tools and treatment plans. This data is generated by algorithms that replicate real patient data, incorporating diverse and representative samples throughout the data generation process.
In finance, synthetic data is used to Model financial scenarios and predict market trends while protecting confidential informationIn addition, institutions can simulate critical financial events to enhance stress testing, risk management and compliance with regulatory standards.
Synthetic data also supports the event of responsive and accurate AI-driven customer support support systemsBy training AI models on data sets that replicate real-world interactions, firms can improve service quality, reply to diverse customer requests, and increase support efficiency – all while maintaining data integrity.
In many industries, synthetic data helps limit the danger of model breakdown. By providing latest datasets that complement or replace human-created data, they reduce the logistical challenges related to data cleansing and labeling and lift standards for data privacy and integrity.
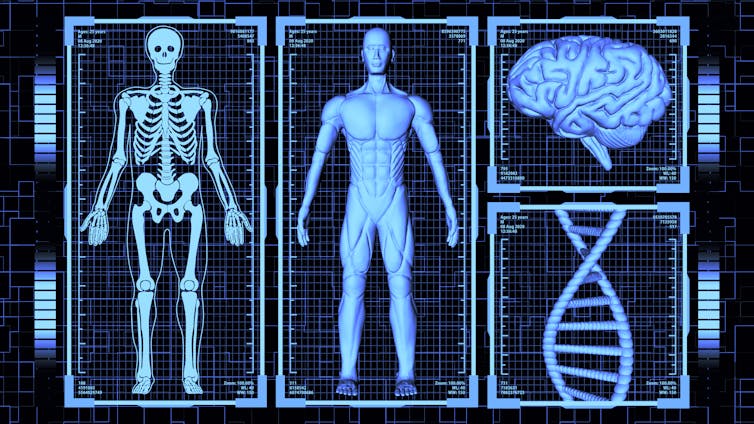
(Shutterstock)
Dangers of synthetic data
Despite its many benefits, synthetic data brings with it some ethical and technical challenges.
A serious challenge is to make sure the standard of synthetic data, which is decided by its ability to accurately reflect the statistical properties of real data while preserving privacyHigh-quality synthetic data goals to enhance privacy by adding random noise to the dataset.
However, this noise might be reverse engineered and poses a major threat to privacy, as in a current study by the United Nations University.
Reverse engineering of synthetic data runs the danger of deanonymizationThis happens when synthetic data sets are deconstructed to disclose sensitive personal information. This is especially the case under regulations similar to the General Data Protection Regulation (GDPR) of the European Unionwhich applies to all data that might be traced back to a person. Although this risk might be mitigated by programming precautions, reverse engineering can’t be completely avoided.
Synthetic data also can Introduce or reinforce biases in AI models. Although it will probably reliably generate diverse data sets, it still struggles to capture rare but crucial nuances present in real-world data.
If the unique data incorporates distortions, These might be replicated and amplified within the synthetic dataresulting in unfair and discriminatory outcomes. This issue is especially concerning in sectors similar to healthcare and finance, where biased AI models can have serious consequences.
Synthetic data also has difficulty capturing the total spectrum of human emotions and interactions.leading to less effective AI models. This limitation is especially relevant in emotion AI applications, where understanding emotional nuances is critical for accurate and empathetic responses. For example, while synthetic data generalizes common emotional expressions, it will probably overlook subtle cultural differences and context-specific emotional cues.
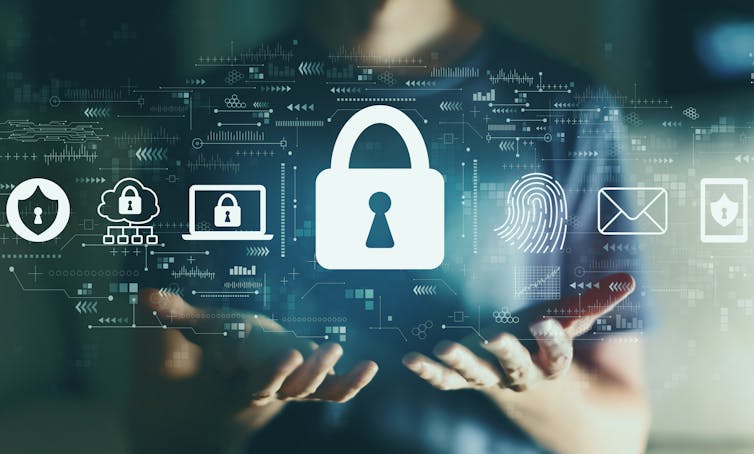
(Shutterstock)
Advancing artificial intelligence
Understanding the differences between artificially generated data and data from human interactions is critical. In the approaching years, organizations with access to human-generated data may have a major advantage in constructing high-quality AI models.
While synthetic data offers solutions to privacy and data availability issues that could cause models to interrupt, over-reliance on it will probably create the very problems it’s designed to resolve. Clear policies and standards are needed to make sure its responsible use.
This includes robust security measures to stop reverse engineering and be certain that data sets are free from bias. The AI industry must also address the moral implications of knowledge collection and the introduction of fair labour practices.
There is an urgent need Go beyond categorizing data as personal or non-personalThis traditional dichotomy fails to capture the complexity and nuances of recent data practices, especially within the context of synthetic data.
Because synthetic data incorporates patterns and features from real-world datasets, it challenges binary classifications and requires a more nuanced approach to data regulation. This shift could lead on to more practical data protection standards that reflect the realities of recent AI technologies.
By governing the usage of synthetic data and addressing the challenges related to it, we are able to be certain that AI advances while maintaining accuracy, diversity and ethical standards.